# Deployments - Model Environments
# Overview
In each Kortical model, models are deployed to a series of deployment environments. This part of the platform is accessible via the cloud icon on the sidebar.
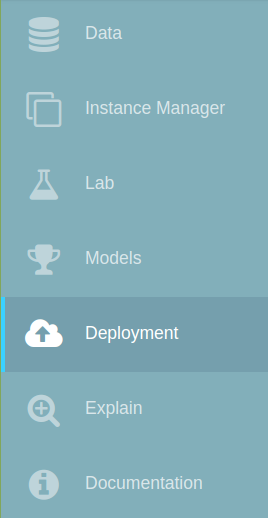
The primary deployment environments are:
- Integration
- UAT
- Production
This is the view the user sees in the platform after they click on the cloud icon.
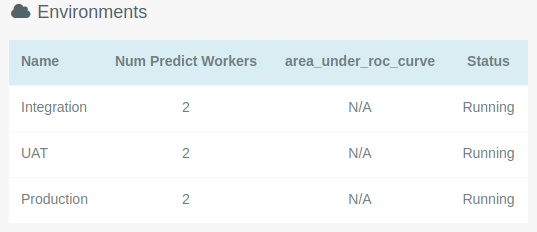
Note
The value of Num Predict Workers
is the number of API workers that serve the predict API for that deployment. This can
be scaled up on demand to increase parallelism and request throughput. Please contact the Kortical team to find out how.
More information about the predict API can be found here.
These names are probably familiar to developers with experience in deploying and maintaining software using different environments. We have applied the same standard practice of separating these environments to deploying machine learning models.
In a given model, each deployment will have a model version 'published' to it. This model deployment (which we will refer to as deployment) is accessible via an API which allows users to send predictions against that model. Each deployment has its own predict API URL, which does not change, regardless of the which model is published to that deployment.
Note
When a model first gets published from the Model Leaderboard page, it is published into the Integration
deployment.
This actually also means that the model automatically gets published to the UAT Ghost
deployment. You can read more on
that in the section about ghost deployments.
For example, the URLs for a model called 'my-model'
for the different deployment could be:
# Integration
https://platform.kortical.com/kortical/platform-demo/api/v1/my-model/predict/integration
# UAT
https://platform.kortical.com/kortical/platform-demo/api/v1/my-model/predict/uat
# Production
https://platform.kortical.com/kortical/platform-demo/api/v1/my-model/predict/production
The idea is that a user can 'mirror' their own software environments that are using a Kortical model (via the URLs) to the deployments in that model.
New models can be published to e.g. the Integration
deployment, without the user having to make any code changes on their side as the URL is static. The same
principle applies for the UAT
deployment and the Production
deployment.
When a user has tested a new model in their Integration
deployment (perhaps this is an improved model that's been trained
with more data than its predecessor), with the click of a button, that model can now be deployed to the UAT
deployment and
then similarly, to the production deployment without the user having had to make any code changes.
Note
Every deployment keeps a record of all previous models that were published to it. It's easy to roll-back to an older model if required.
# What's in a deployment?
In this section, we will examine what a deployment looks like in the platform UI and what features are available. This is a deployment page. Specifically, this is the
Integration
deployment in a model called titanic
.
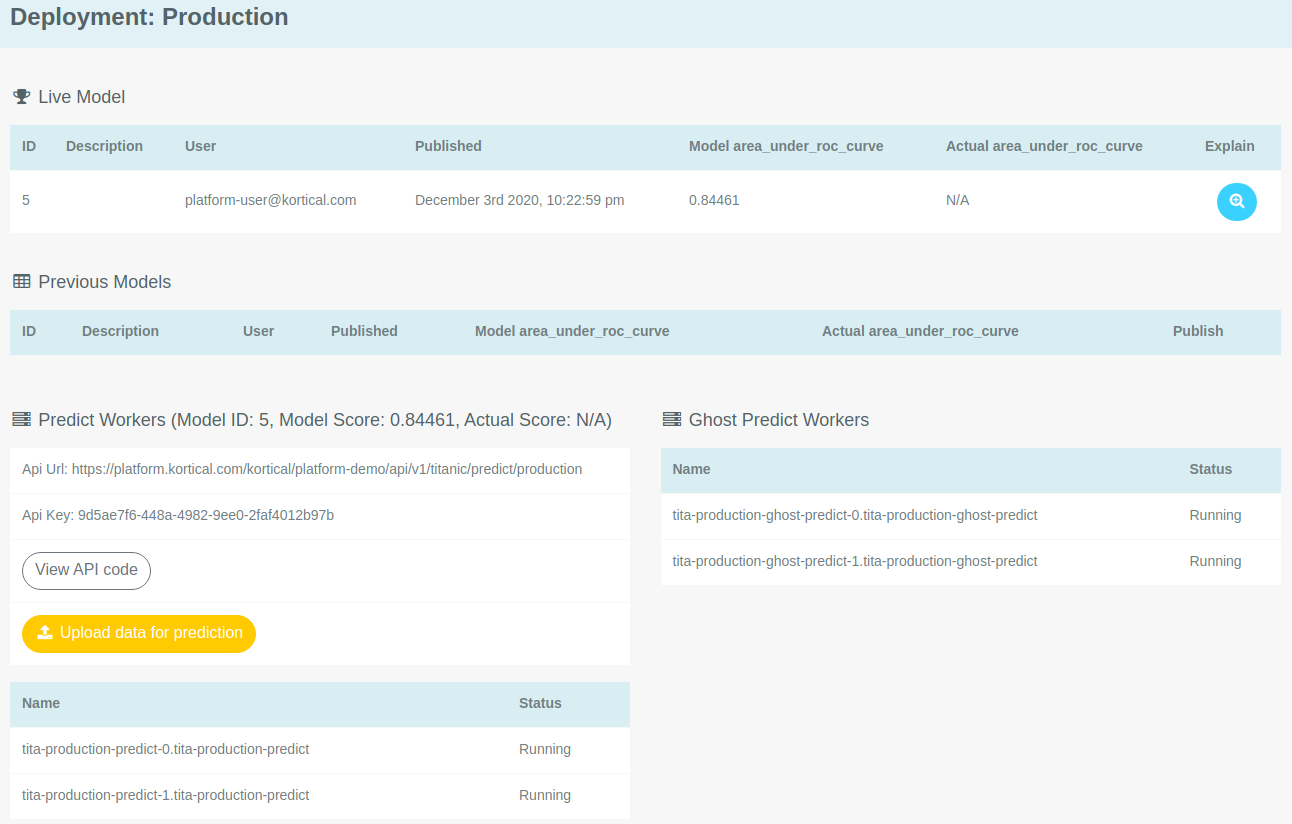
The Live Model
section displays the model that is currently deployed to Integration
, along with some information about it.
The Previous Models
section displays the models that were previously published to this deployment. These can be re-published using the Click to Publish
button
on the right of each row.
The section at the bottom, under Predict Workers
contains the URL for this deployment as well as some other functionality.
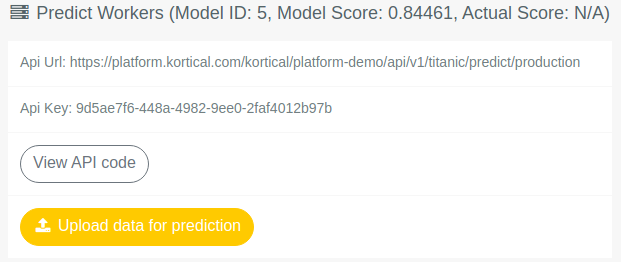
The Upload Data for Prediction Button
allows the user to upload a CSV file that matches the models expected input. This will return a new CSV file with all the
row predictions that will be downloaded to the users machine from the browser.
# Deployment API Page
The View API Code
button will take the user to the API page for a given deployment.
# API Playground
The API Playground is an utility that lets the user send requests to and experiment against the predict API for a given deployment. This is explained in detail here.
# Code Snippets
This section contains ready-to-use code snippets and Google Sheets integration to get predictions from a given deployment.
# Ghost Deployments/Publishing models
A 'ghost' deployment mirrors the deployment that is directly 'below' it. In the Kortical deployments, Integration
is directly below UAT
and UAT
is directly below Production
.
Therefore, there are two Ghost deployments in a model. These are:
UAT Ghost
- mirrorsIntegration
Production Ghost
- mirrorsUAT
So this means that if a model is published to Integration
, UAT Ghost
will be updated automatically with the same model. Same goes for UAT
and Production Ghost
.
These Ghost deployments exist in case a user wants to monitor e.g. how the Integration
model would do if it was running in UAT
. That would be where the UAT Ghost
deployment would come in.
In the image below, UAT Ghost
is circled. The model that is currently published to the Integration
deployment has ID 6
, therefore the UAT Ghost
deployment also has the same model.
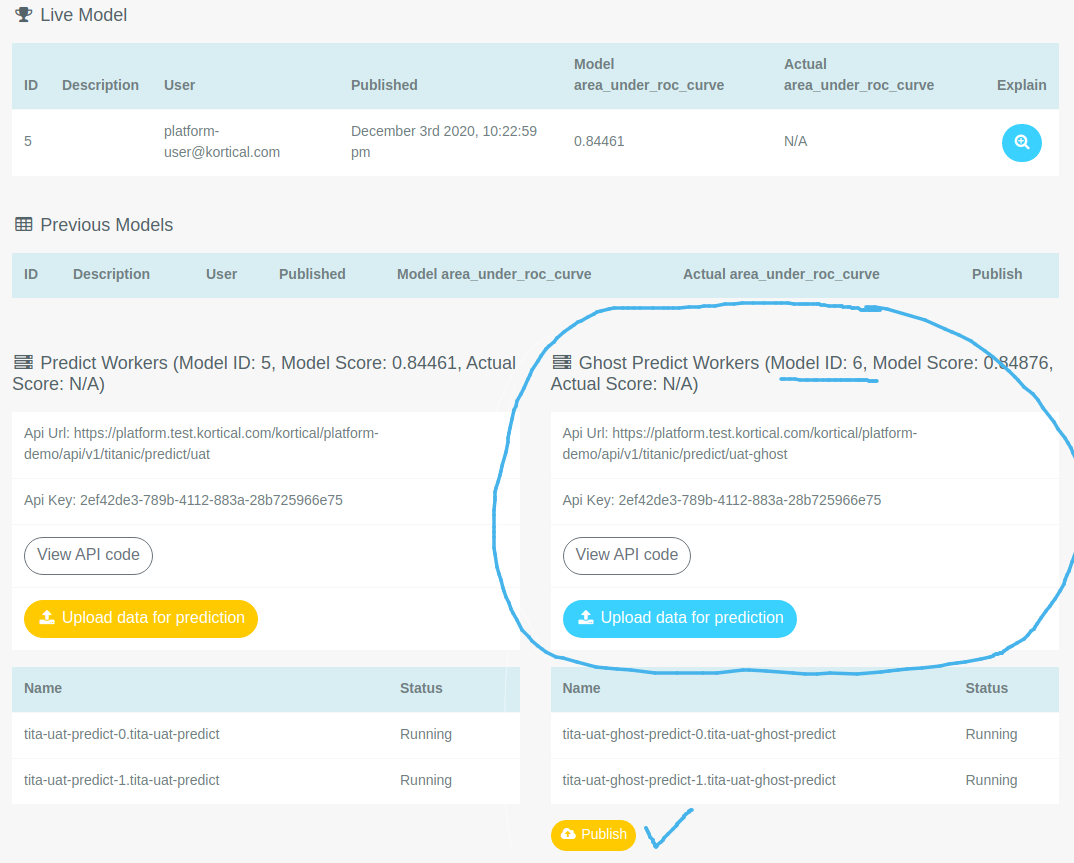
If we click the Publish
button, which is next to the blue tick, we get a pop-up asking us if we're sure that we want
to publish this model to the UAT
deployment.
Note
There is no downtime when a new model is published into a Kortical deployment.
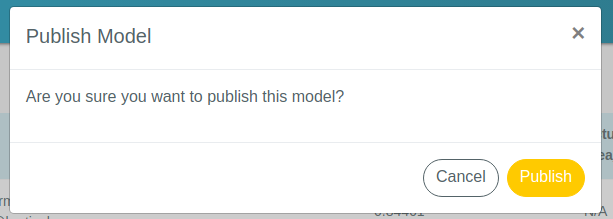
If we select Publish
, then the model with ID 6
will now be deployed to the UAT
deployment.
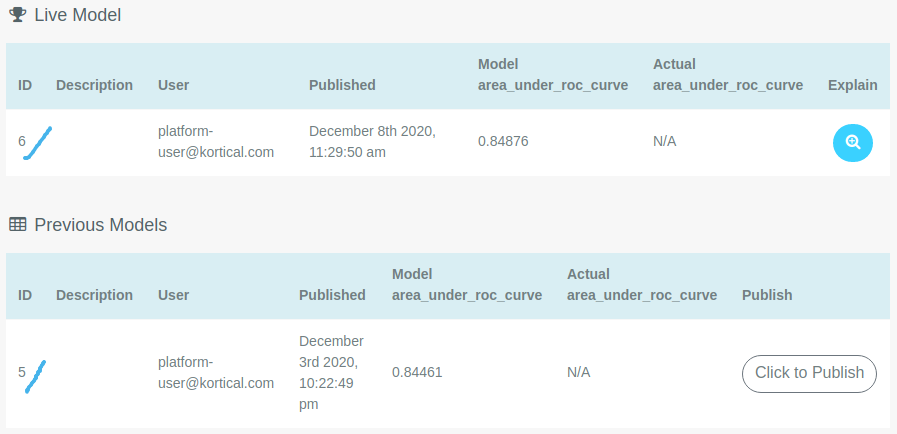
Now the live model is model 6
and the model that was there previously, model 5
is listed as one of the previous models.
Note
Publishing model 6
into the UAT
deployment now means that model 6
is also currently active in the Production Ghost
deployment.