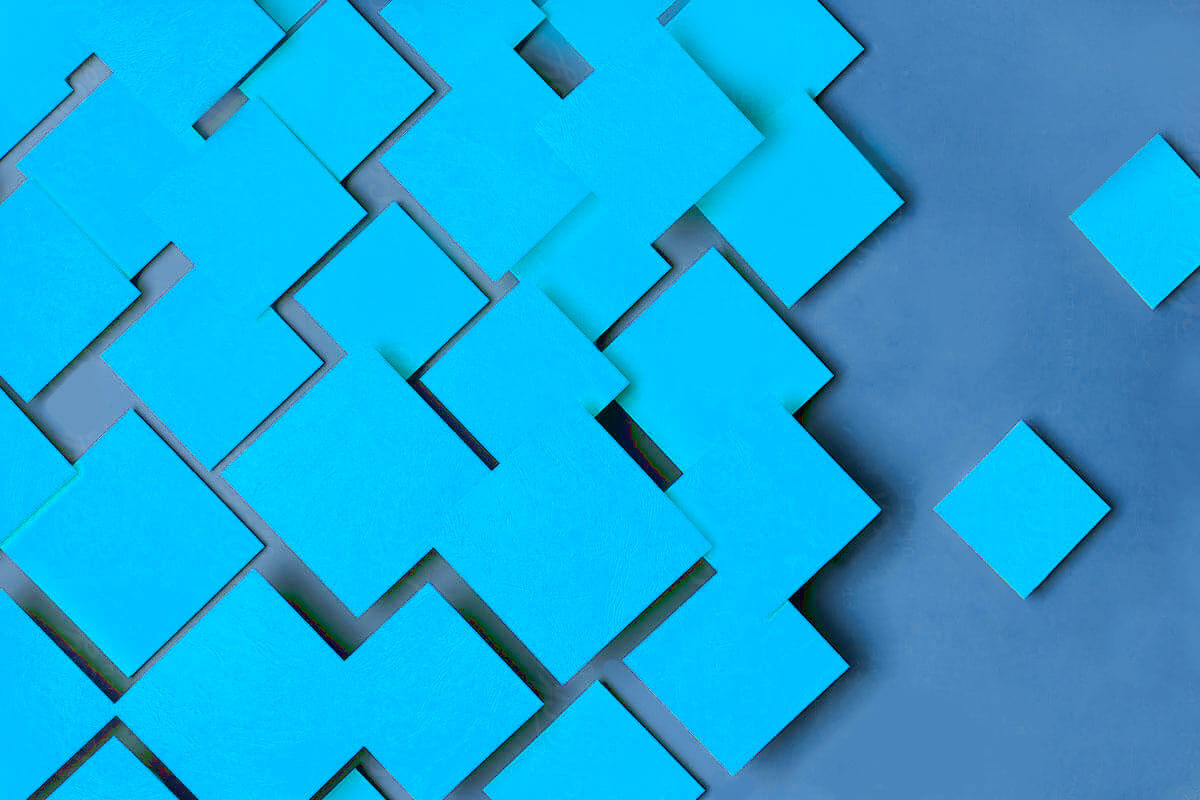
With so many “as a Service” offerings springing up it can be hard to keep track but AI as a Service (AIaaS) is gaining popularity with businesses as they embark on their AI development. Allied Market Research that annual global AIaaS revenue is forecast to grow from $2.38 billion in 2017 to $77.05 billion by 2025, taking it from niche to mainstream in a few years.
Simply put AI as a Service allows companies to in effect rent all the component parts you need to create best in class AI models and deploy them easily, to get value out of the organisations’ data fast, with much less risk.
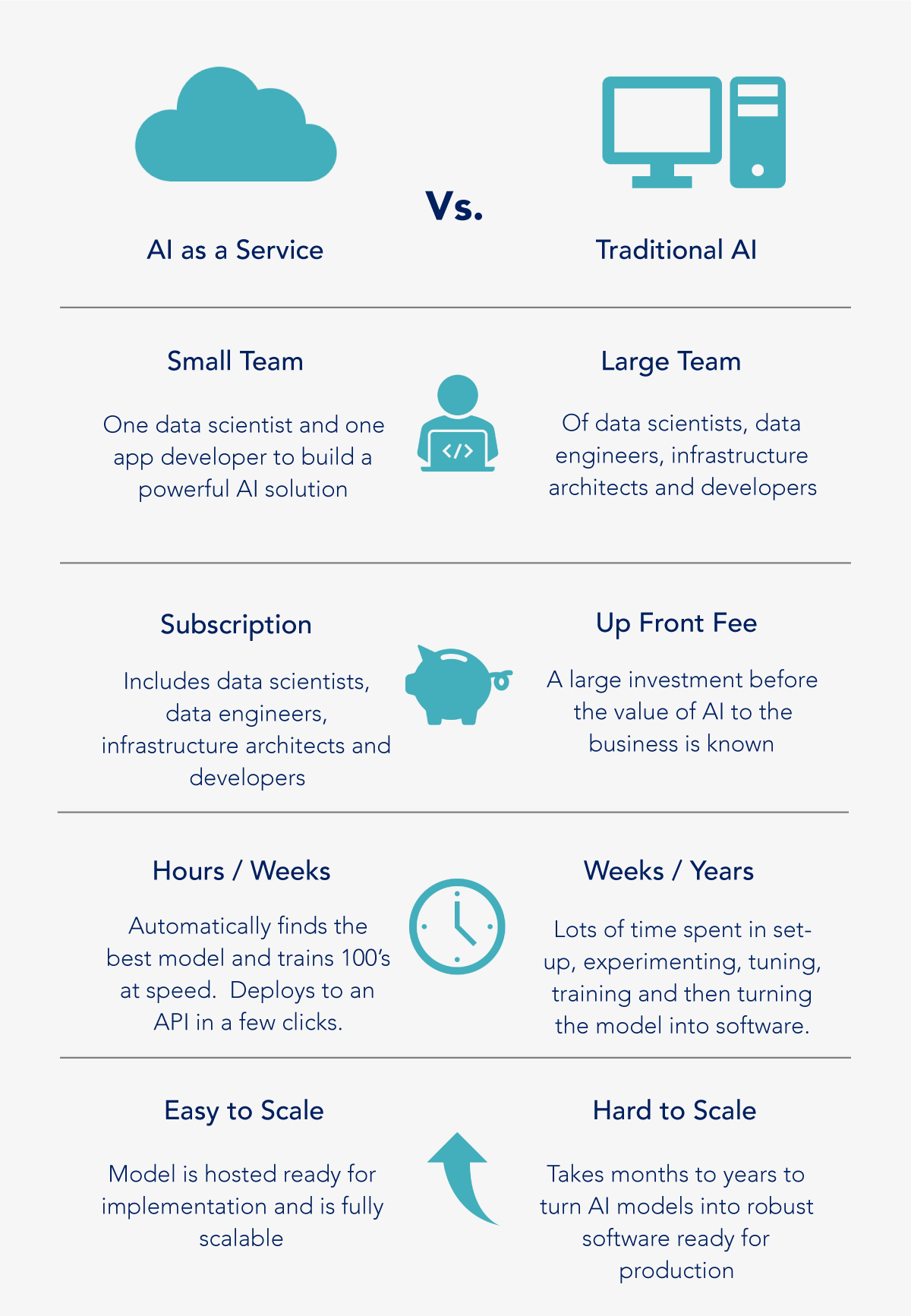
Traditionally businesses would have had to hire a team of data scientists, researchers, data engineers and software developers as well as build or procure their own cloud or hardware to be able to handle the compute power required to train models. Even when these businesses feel they have the best model, they might still spend months or years working on turning it into software that works at scale to the SLAs that the organisation requires.
This has typically meant huge investment without first being able to try things out or experiment with the data and this has resulted in AI getting a bad reputation at times.
An example of something you would want to know before investing in a large AI team, would be if the best model is going to be 60% or 90% accurate? Potentially at 60% it is not worth investing in that solution as the business case might not make sense at this accuracy level and you should spend time investigating another problem instead.
Without an AIaaS tool you can imagine how costly that one experiment could be.
The typical AIaaS platform (which is also at times called MLaaS) has the latest AI/ML techniques already built into the system and some also have the cloud and infrastructure part taken care of too. This means it is simply a case of plugging in your data, hitting train and letting the platform build features, find the best model and then tune its hyperparameters.
They often have model explainability which means you can see the features that are driving the decisions.
Explainability is key for many regulated industries in which organisations are not allowed to have a black box model making decisions for them.
A commonly overlooked question of any AI model/solution is how good the results are, as in business, results can really matter. If you currently have people reading emails or making tax decisions and you would like this to be automated by AI, the decisions the AI makes need to be as good or even better than the humans who are currently doing it.
Whatever AIaaS you are considering, make sure accuracy of the results are tried and tested on business datasets.
A great AI model is worth nothing by itself, it needs to be deployed as part of a product or app. Deployment often takes a lot longer than estimated to turn the AI model into a service ready to be consumed by an application or website at scale. Often AIaaS solutions take care of the deployment process and host the models in a cloud like way which means it can scale up to millions of real time interactions while keeping latency low.
The beauty of some of the AIaaS platforms is that they also automatically retrain new models as new data becomes available, ensuring the model integrity is high and that you will have consistently high performing models with little manual input.
Once you have an AI solution that is performing at a high level, the last thing that you want is to tie your top talent into its maintenance. Additionally, as we add more data, a totally different model type might become the most optimal so having a solution that can do the data science work of model selection work as well as taking care of the retraining takes a huge amount of time away from the model’s ongoing maintenance costs while still keeping performance cutting edge.
While some businesses require only one AI or ML model to get great value, often you need two or more models working together for more nuanced problems and this is another area where AIaaS solutions can be very beneficial.
Due to the fact that these solutions automatically find and build you the best models vs having to manually experiment with different models and then waiting for each of those experiments to run, the ability to have parallel experimentations running in one session means that you can easily keep building the best AI/ML models, ensuring that you keep building more and more value for your organisation.
In Summary
While of course you can hire the big team, spend time on piecemeal open source solutions and then write and test all the code to manage the whole operation end to end manually, the question is could AI as a Service be a more efficient use of your time and budget? AI as a Service lets you spend the majority of the time and budget solving the business problem, not trying to build an AI research and operations capability that will then get you to a place where you can then try to solve the business problem.
AIaaS enables businesses to fill skill and technology shortage gaps, experiment at speed and reduce ongoing operating costs.
This is why Magnus Revang, research vice president at Gartner, states that “by 2023, over 50% of enterprises will be using AIaaS to enhance their application portfolios, up from less than five percent today. I’m excited to see what this future looks like.”
Do get in touch if you would like to hear how the NHS, BT and more have taken advantage of the benefits of AIaaS.
Get In Touch
Whether you're just starting your AI journey or looking for support in improving your existing delivery capability, please reach out.
By submitting this form, I can confirm I have read and accepted Kortical's privacy policy.