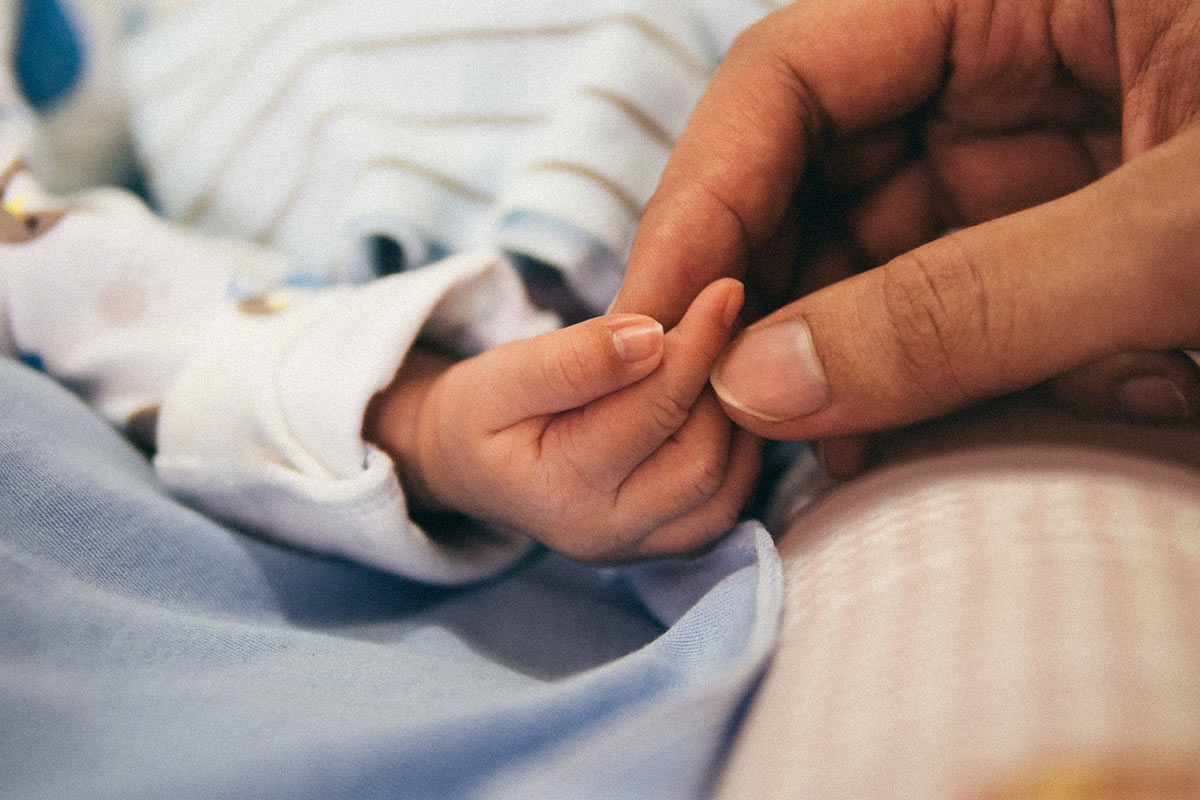
AI supply chain optimisation for platelets to reduce costs
54%LESS EXPIRES
100%LESS AD HOC TRANSPORT
06MONTH FULL DIGITAL TRANSFORMATION
The speed of results and very high levels of accuracy achieved by Kortical through the deployment of the Kortical platform were impressive.
We met some forward thinking partners at Deloitte, who were open to working with a start-up and upon seeing the Kortical platform understood how impactful it could be for their business. We started with a 4 week POC on a limited dataset on the most crucial part of the process, to prove out the level of accuracy / automation achievable and thus be highly indicative of the ROI.
Key factors in selecting Kortical were:
Over the years Deloitte has refined and optimised the set of steps for this particular tax process, to ensure that the highest levels of quality are achieved, while also being cost effective. We worked with senior stakeholders to map this process focusing on where and how it would make sense to apply AI / ML to greatest effect.
The key tenets we keep in mind when defining AI strategy are:
Once these have been mapped, the optimal AI roadmap becomes very apparent. In this case the two biggest wins were in the automated application of tax law and in automatically structuring unstructured client data, with a number of ancillary applications for ML in the process.
Great machine learning models require a combination of data, deep domain knowledge, data-science capability to map the domain to machine learning effectively and AutoML / Cloud scale to find the best solution possible.
As the problem is better understood, the domain experts are tweaking and refining the training data and the data scientists are figuring out new better ways to represent this data for machine learning. Because of this, the best feature generation, NLP techniques, model to use and hyperparameters for all of the above to get the best results, constantly evolves. This is where having great AutoML comes in, by having the best in class tool for this, the data scientists are focused on high level feature engineering rather than spending time laboriously searching through alternative models and parameters that are hard to intuit.
Kortical uses cloud scale AutoML and given the volume of training data Deloitte have, this is a crucial advantage, rather than taking 32 days as it would on a single machine to create a new high performing model with all the right parameters, we can scale up the compute and number of automated workers to ensure rapid iteration and minimal downtime for the data scientists. We found 12 parallel workers brought it down to 2.5 days per model, which was a nice compromise between minimising data scientist downtime and compute costs.
Combined this means that the data scientists explore thousands of modelling approaches and hundreds of high level features in a very short time-frame and as the data and features evolve so does the solution automatically. This rapid iteration makes building world class models much more cost effective and leads to much better outcomes.
Machine learning models are not consumable directly by users, to use these models they need to form part of an app or service. Deloitte has skilled app developers with domain knowledge that built the application to house the machine learning and these were able to call into Kortical via REST APIs to add the machine learning intelligence.
Machine learning models need to constantly evolve and learn as users interact and correct the machine learning. Working collaboratively with Deloitte we helped design a workflow that allows for the AI to automate as much as possible but also allows humans to interact and correct the machine learning outputs where machine learning is unsure in such a way that the corrections can be used to retrain the model.
AI makes predictions -> User corrects or accepts with low confidence predictions - > data fed back into Kortical -> AutoML -> Business defined acceptability tests -> deploy -> back to predict
In this way we can create fully automated self learning AI but with full reproducibility and testing before the models are updated.
Industry is just starting to wake up to the challenges of MLOps (Machine learning operations), the deployment and maintenance of machine learning models. By our estimations this is a large chunk of why Gartner industry surveys show that most organisations expect to have a machine learning solution live in less than 1 year but on average take 4 years to get to live, as people tend to underestimate the challenge.
Deloitte are using complex machine learning models at scale, they require:
A key tenet of our collaboration with the Deloitte Tax team is that we’re helping them to upskill in the use of AI enablement tools like Kortical, so that they can produce their own models and IP.
To get the best possible results it really is a marriage of domain expertise, data-science skills and Kortical. We worked with their domain experts and data scientists, sharing best practice, processes and methodology and their data-sience team is now creating world class models, at speed, on Kortical, independently.
AI and machine learning are not a commodity. Better models, means improved ROI. In this case it means more automation and less human intervention required, which translates into cost savings where the human can do more valuable work.
The scope of the project was very ambitious, using machine learning to automate end to end from raw unstructured data, through to processed accounts but with the controls in place so humans can oversee, correct and teach the AI. To accomplish this required many machine learning models working together to automate various aspects of the workflow, as well as a UX created by Deloitte that tied it all together. The result took processing from 5 hours to 6 minutes, an incredible 50x productivity improvement. All while maintaining human level quality.
These results were achieved in a 6 month build, followed by User Acceptance Testing (UAT) and deployed shortly after. This 8x faster than Gartner averages for AI / machine learning solution deployment, while also being at the upper end of the scope / complexity of AI projects.
Whether you're just starting your AI journey or looking for support in improving your existing delivery capability, please reach out.
By submitting this form, I can confirm I have read and accepted Kortical's privacy policy.