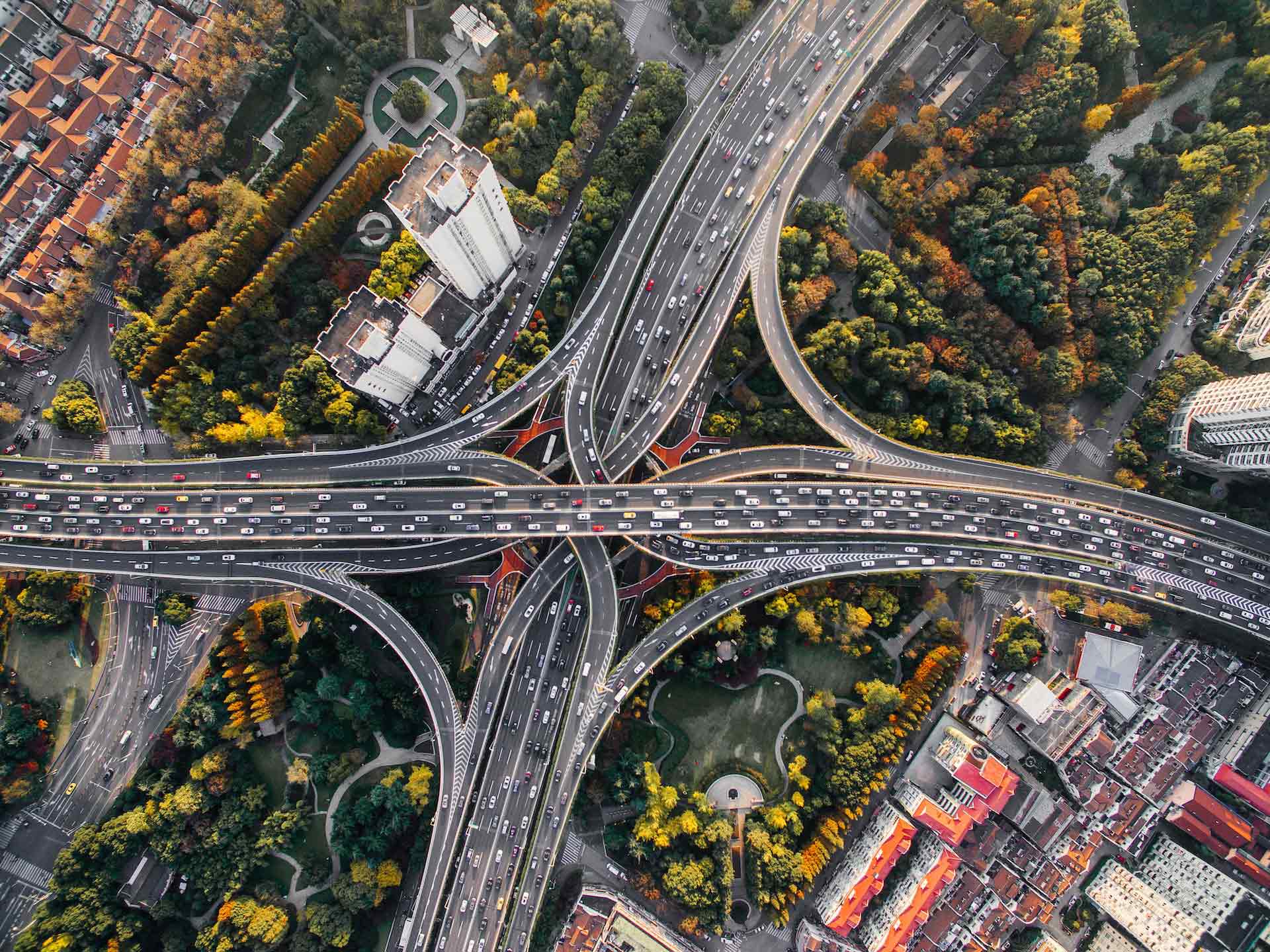
Build better AI solutions with an AI roadmap
Tried and tested framework to find the fastest route to value
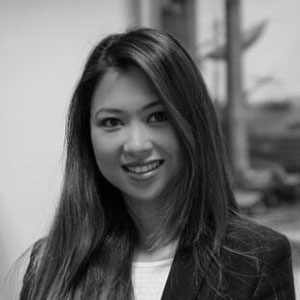
Director
If you're looking to unlock the potential of AI technology for your business, we have found the best place to start is an AI roadmap to identify what we call a "lighthouse" use case, the best AI solution to start with that will have a big impact and show the wider organisation how valuable it can be, fast. Once you have found this use case and proved its value, you can attract more investment and get people on board to unlock more artificial intelligence resources and projects.
In this article, we'll go through how to create an AI roadmap and the components involved to set you up for success.
Table of Contents
- The regular software approach is not the same for AI solutions
- AI Approach: find the Lighthouse use case
- Roadmapping session
- Steps to creating an AI roadmap
- Step 1 - Discovery - a 1st principles approach
- Step 2 - Create a list of AI applications
- Step 3 - Score the use cases
- Step 4 - Map out to visualise AI solutions
- Step 5 - Rank your use cases
- Conclusion
The regular software approach is not the same for AI solutions
Surely, a regular software framework would be a good start right? We've had over 20 years to refine this framework to build digital technologies and tools that augment human capabilities.
Unfortunately, a regular software approach will not work entirely when building AI solutions. The challenges and complexities of working with artificial intelligence require a different type of planning and execution.
The thing is that when we think about software roadmaps, what we've often seen is that they're talking about how to pass data from one place to another or how to manage data moving around. For example, if you want to improve communications with a bank, you build an app to get the data from here to there in a nice structured format and workflow system.
What's different about AI and intelligent automation use cases is that often, they're trying to replace an existing process. But you can't be sure that automation will work and you might not get a sufficient amount of accuracy to make it worth the time and money you've invested.
With AI, there are a lot of subtleties involved with data and figuring out if it will work well enough so it takes a lot of experimentation and iteration, something you don't always get with regular software development projects.
AI Approach: find the Lighthouse use case
We see that a lot of organisations start with huge, grandiose plans for AI transformation that involve a lot of change management. This can get bogged down in politics easily. This is almost borne out of the fact that Gartner says about only 15% of AI projects succeed. So there are quite a lot of people who are rightfully skeptical of AI - senior people or those on the front lines who have to work with these systems or implement them successfully.
Because AI is seen as experimental, there may not be that much funding for it initially. The goal is to find the lighthouse use case, one or two AI use cases where you can dive in and create a lot of value quickly and make everyone in the business wake up to realise that AI is going to have a positive impact. You need to find the use case with the highest likelihood of success and at the same time yield considerable value.
When you get ROI from those first few use cases, it will almost be guaranteed that you'll do more because you've proven the value of the first one. You start building the AI innovation function from there.
Roadmapping session
Who should be in the room
A roadmapping session should include the sign-off stakeholder, domain expert, data expert, and AI expert.
The sign-off stakeholder
The sign-off stakeholder needs to be on board with the project to approve the budget and ensure its success. He will be able to answer questions and make key decisions such as what is to be done, how it aligns with strategy, and what business value it will bring.
The domain expert
The domain expert is the person who understands all the business processes across the business in detail. They can help identify what processes may be automatable or what could be augmented. This could also be a number of specialists e.g. someone in marketing that understands how churn, personalisation, ad spend is allocated and another in customer services that know about customer enquiries, staff challenges etc..
The data expert
The data expert is the one who knows where the data is stored, what data is there and how to get access to it. One of the biggest blockers of AI and enterprise is that the data exists but you can't get access to it. Someone who represents all those challenges can help with that issue.
The AI applications expert
An AI applications expert is a term we invented and describes someone who can bridge the worlds of business, AI and machine learning. This person should be able to quickly internalize the business context, processes and data expertise and propose how to apply AI across the various business functions to generate value.
While the typical AI expert is an academic who can do complex linear algebra as simply as one might tie their shoe laces, they often don’t have a deep knowledge of how businesses function or much experience of successful AI deployments in industry. The AI applications expert embodies the marriage between a business consultant and deep technical knowledge with hands on experience of delivering AI projects. The two keys being:
- Someone who has deep experience of deploying AI across a wide range of use cases in industry to provide returns and as such can spot the opportunities.
- Someone who understands machine learning at a deep enough level that they can intuit the significance of differences in the data and business processes and the impact that has on the applicability of various possible AI solutions.
Having an AI applications expert on board will help ensure that businesses are optimising for the right outcome and not just chasing metrics that don't necessarily align with the end goal.
Steps to creating an AI roadmap
Watch the video below where we role-play how we would go about creating an AI roadmap for a generic retail company.
Step 1 - Discovery - a 1st principles approach
Get a deep understanding of the end-to-end processes for main revenue and cost drivers to identify where you can apply AI to further improve its efficiency and optimise the process.
The main revenue drivers for a company can include factors such as sales, marketing, and customer service. The main cost drivers can be things like employee salaries, materials, facility costs, and equipment expenses.
Step 2 - Create a list of AI applications
Based on the processes, list down where you might apply AI. For example:
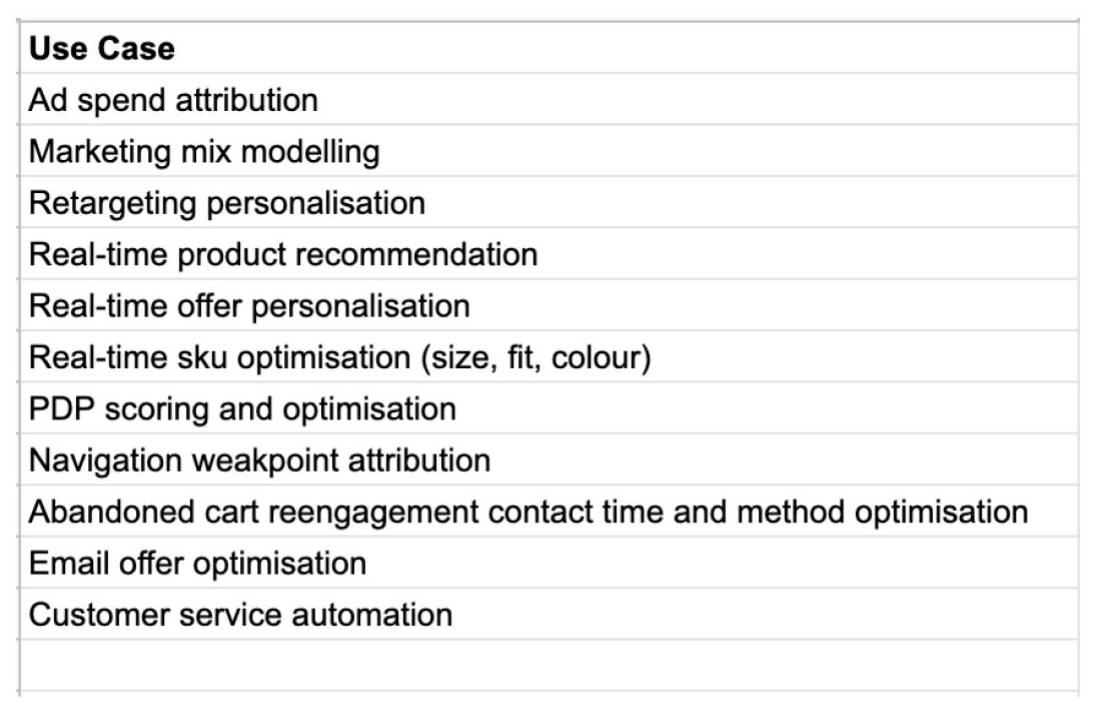
Step 3 - Score the use cases
Work with everyone in the room to score for business value, strategic alignment, solution complexity and data complexity.
- The domain expert and sign-off stakeholder will give scores for business value and strategic alignment.
- AI experts will give the score for solution complexity.
- The data owner who understands how hard it will be to get access to the data will score for data complexity.
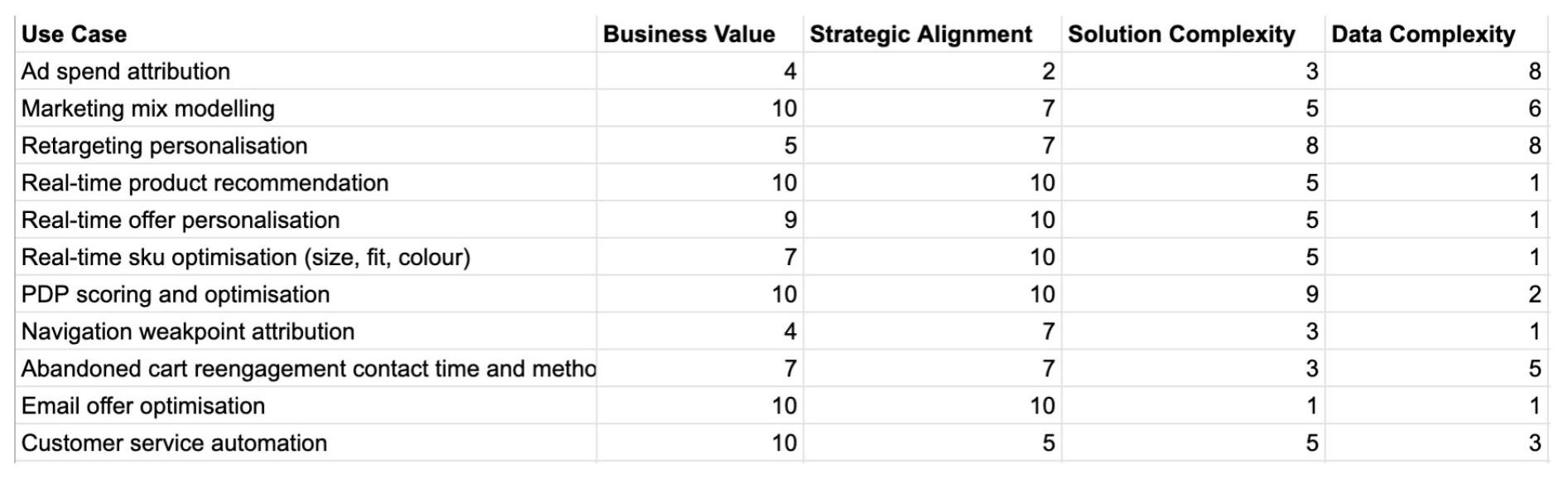
Step 4 - Map out to visualise the AI solutions
Create a simple 2d projection to have a visual representation for the use cases.
We use this simple formula:
X = Business Value + (Strategic Alignment / 2)
Y = Solution Complexity + Data Complexity
X - Actual real material value is more important than strategic alignment by some modification factor, you can choose whichever you want but in this case, we choose 2.
Y - The complexity of the solution plus the data complexity is basically how long it will take to get the data and build the solution.
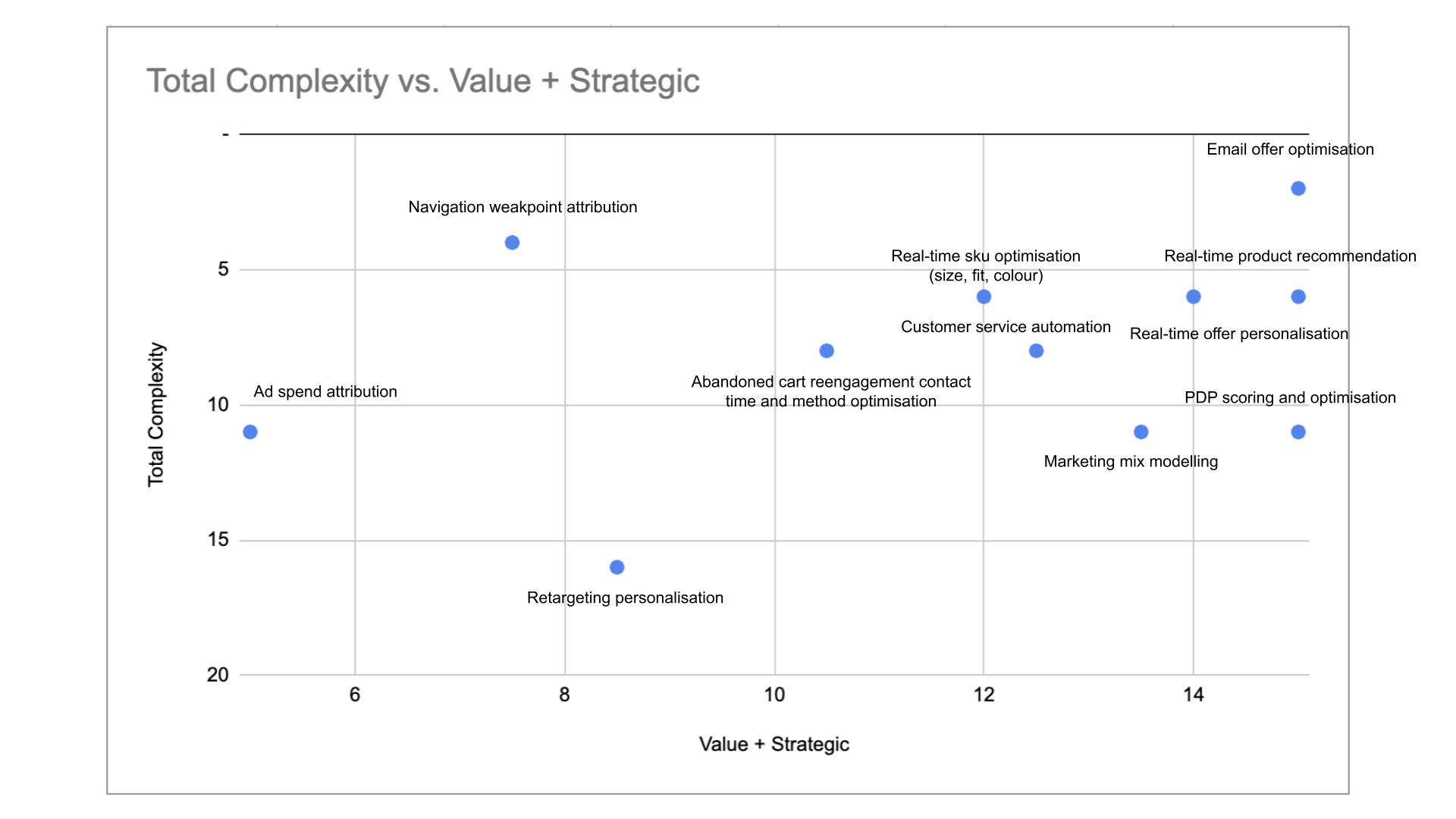
The lighthouse use cases, those with low complexity and high value would be those on the top right (area right of the pink line).
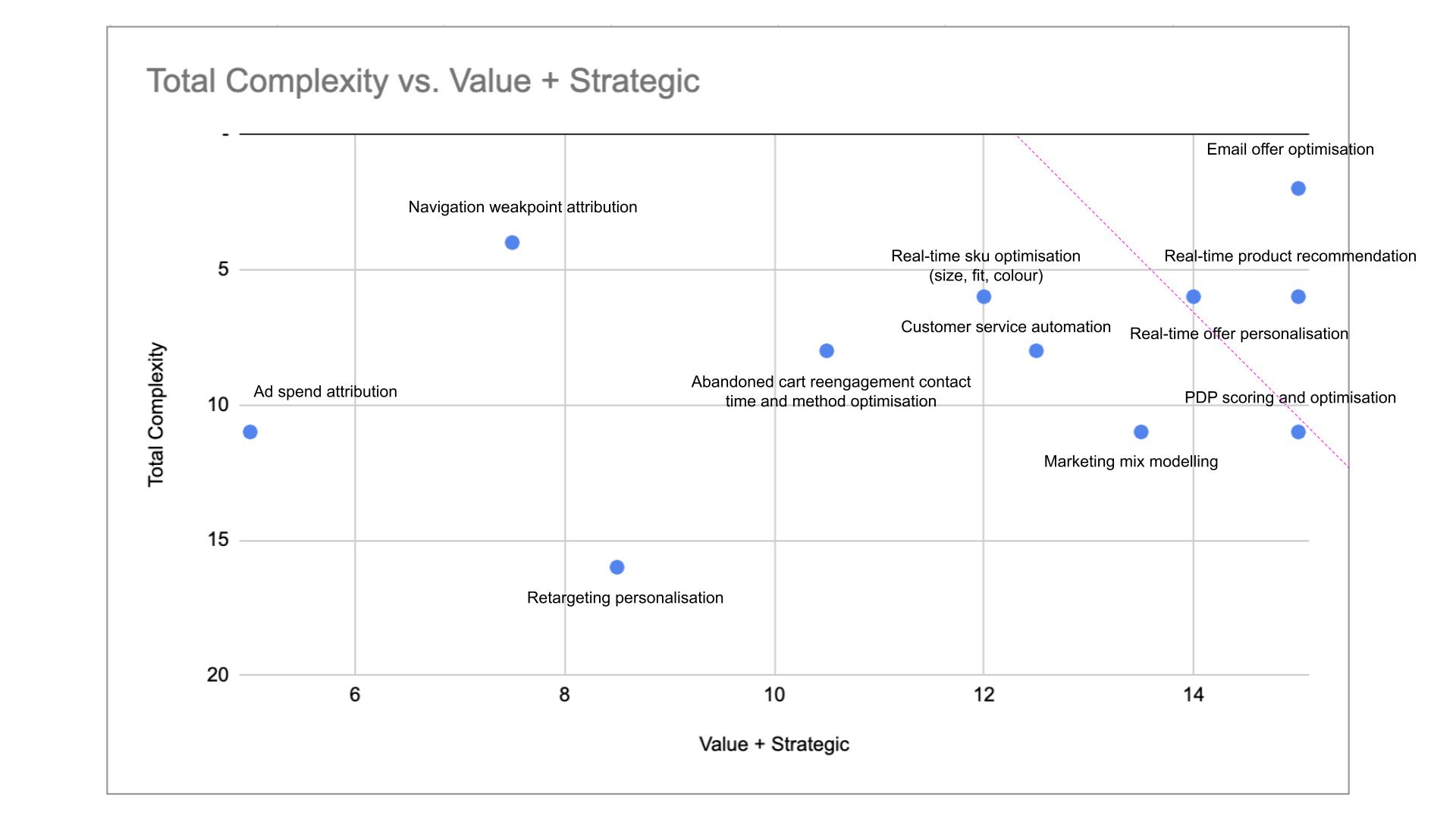
Step 5 - Rank your use cases
Simple Formula:
Rank = -x(Value + Strategic Alignment) + Y(Complexity)
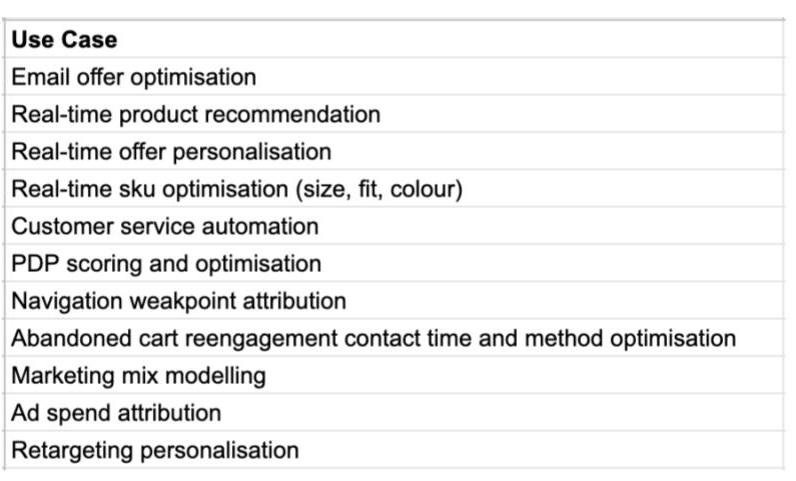
Free Download
Conclusion
There is no hard rule on when you should or should not go about creating an AI roadmap. You can create one at any point in your digital transformation or artificial intelligence journey. At times, the use case is obvious so an AI roadmap is unnecessary. It is often the case that clients, usually at stakeholder levels, who come to want AI for more pressing, tactical issues have specific use cases they want to validate that there is no need for an AI mapping exercise.
However, the times we've seen AI projects fail is when we get involved after they have determined the AI project they want to do. They think the AI use case would be great but they haven't looked at what data is available.
Data is the key to success for AI projects and if a company doesn't have the data or the data is collected but the way it is structured is not suitable for AI, the project will likely fail. Bringing in somebody who knows about AI as soon as possible can advise on the appropriate data collection and structure for AI applications.
To conclude, there is no one-size-fits-all answer when it comes to whether or not a business should create an AI roadmap but if there is an opportunity to do more than one thing, question whether or not that's the right thing to do right now. If you continue without exploring the options, you can get trapped in a project that is not successful or as successful as it could be.
Machine Learning podcast series: Build the AI future
We love Artificial Intelligence (AI). We believe that AI is a force for good that can benefit everyone. The AI future has already started and is changing how everything gets done.
"~50% of all current work activities are technically automatable using currently demonstrated technologies." - Mckinsey (link)
However, so many AI projects fail - about 85% according to reports from Gartner yet the outpour of investments in AI technologies remains strong.
This podcast series shares our learnings and experiences in delivering AI-driven digital transformation for Fortune500 companies like the NHS, Deloitte, Ford and start-ups like Charlotte Tilbury and flydocs. Our goal with this Machine Learning series is to provide insights into business processes and practical tips from real-world examples of AI that showcase how to successfully deliver AI/Machine learning solutions and build towards the AI future.
Check out the other podcasts here.
About Kortical
Kortical is an enterprise AI cloud platform that provides any company with data-driven insights, model building, deployment and ML management infrastructure solutions.
We are strategic partners for AI, empowering some of the world's best companies to deliver high-value machine learning solutions, quicker and of better quality, than they were able to before.
We’ve delivered real world AI and use cases. Here are some examples:
Industries: Retail, Healthcare, Telecoms, Finance, Manufacturing, Consulting
AI Techniques: Binary Classification, Recommendation, Optimisation, Ensembles, Forecasting, Regression, Time Series, Sequence Classification, Multi-class Classification, Transformers, Natural Language Processing (NLP), Multi-label Classification, Deep learning
Get In Touch
Whether you're just starting your AI journey or looking for support in improving your existing delivery capability, please reach out.
By submitting this form, I can confirm I have read and accepted Kortical's privacy policy.