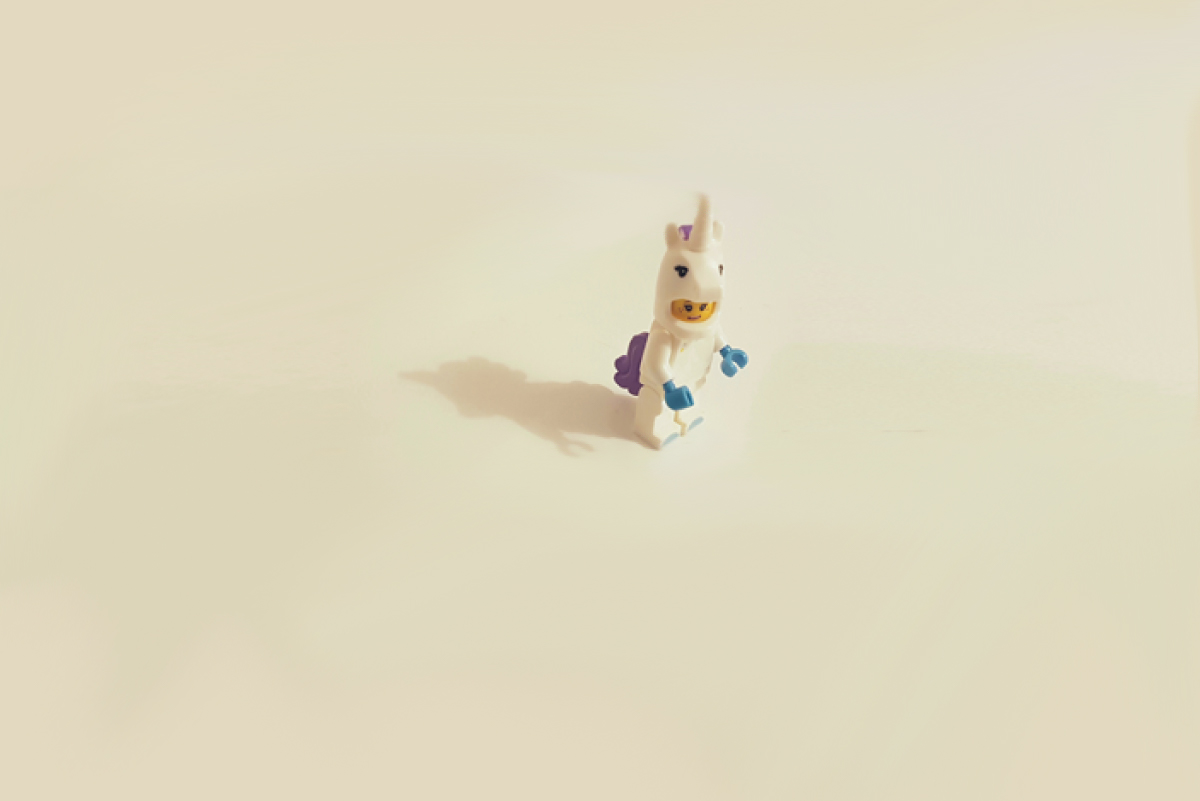
The Myth of the "Citizen Data Scientist"
What it really takes to deliver business value with AI / ML
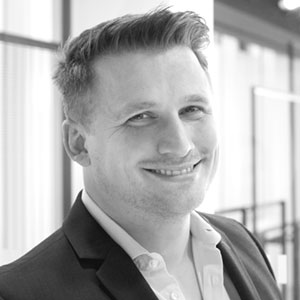
Co-Founder, CEO & CTO
With advances in the branch of AI that is using AI to create bespoke machine learning solutions, sometimes known as AutoML there is a lot of talk of the “Citizen Data Scientist”. Which means any person suddenly made data scientist by using AI tools and services such as our platform Kortical. Some of our competitors have run ads touting cucumber farmers as great citizen data scientists. While AutoML tools do empower non data scientists to realise the benefits of AI, you still need some data science capabilities to realise the full benefits. This article aims to debunk some of the hype and tell you how to get the most out of AI and ML no matter your skill level.
Business and Data Analysts
The people who tend to know what their data means to the business inside and out, are often not the data scientists but more business aligned folks. Getting the power to experiment with ML models and trying new approaches themselves is hugely valuable to any business and can rapidly accelerate value extraction. With AutoML platforms like Kortical, this has never been easier and can be a huge boon. The challenge for these users is that there are a few simple and not so simple common mistakes, such as “leaking a class variable”.
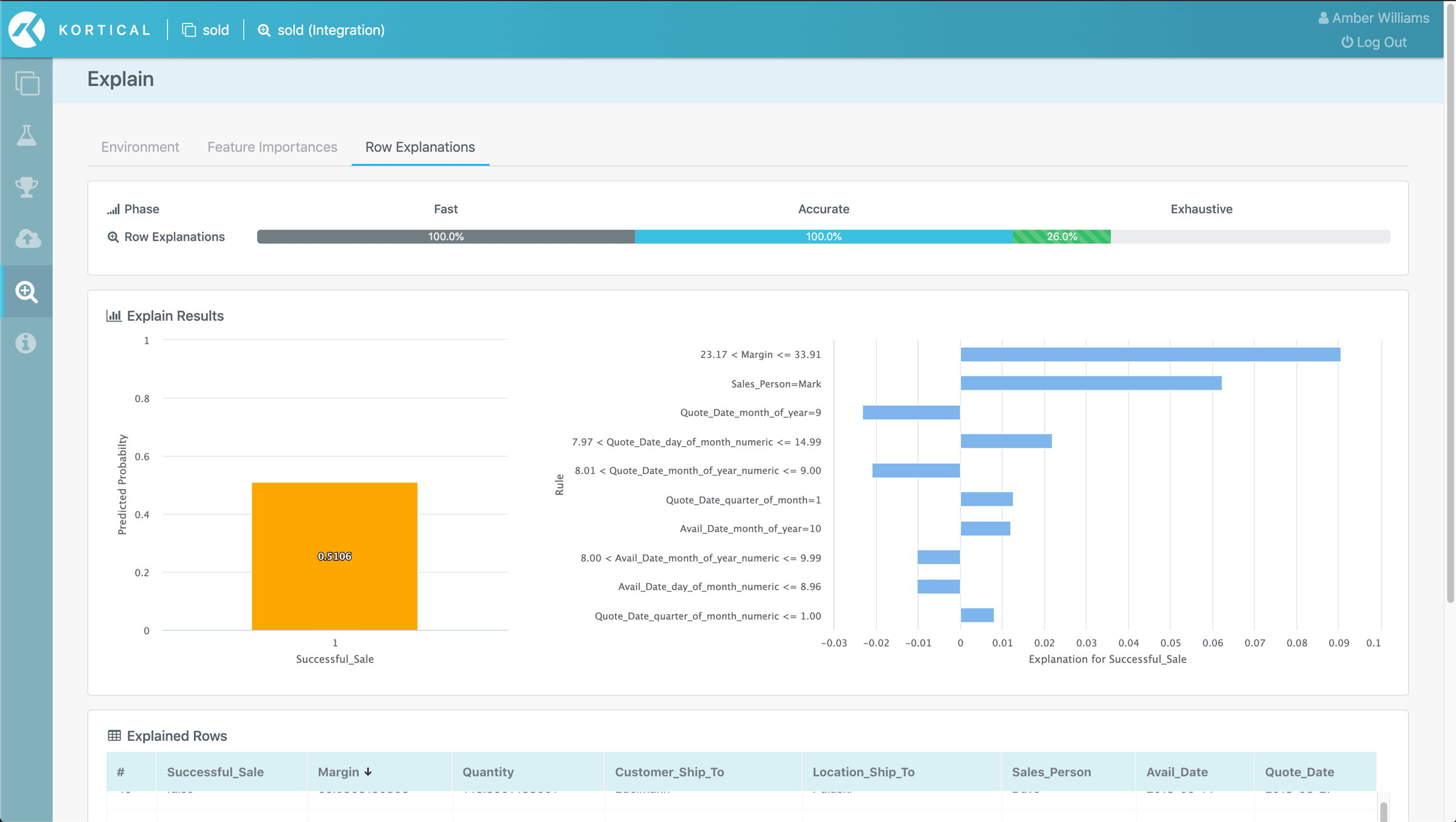
An example might be if we’re building a model to predict if a sale was successful or not, where expenses are one of the variables and it’s tradition to buy a few rounds if a deal is closed. The machine learning might pick up on this and use this to predict a sale, even though it’s only filled in after the fact. In practice this would mean the model would underperform expectations, because it would overweigh the importance of low expenses. To fix this, we could simply remove the expenses variable from the model inputs or subtract out the cost of the drinks when building the model.
How to overcome the challenges
The fix is actually pretty simple, a few minutes with an experienced data scientist is often enough to course correct and allow business users to harness the power of machine learning. For smaller businesses, this might mean booking in a slot with our team to review your models before you put them live or use them for critical decision making. For enterprise, it more likely means putting a governance framework in place with a centralized team of data science capability that teams can draw upon and a review process before models are put into production or used for decision making. With time and experience business users can learn to become proficient in ML model creation.
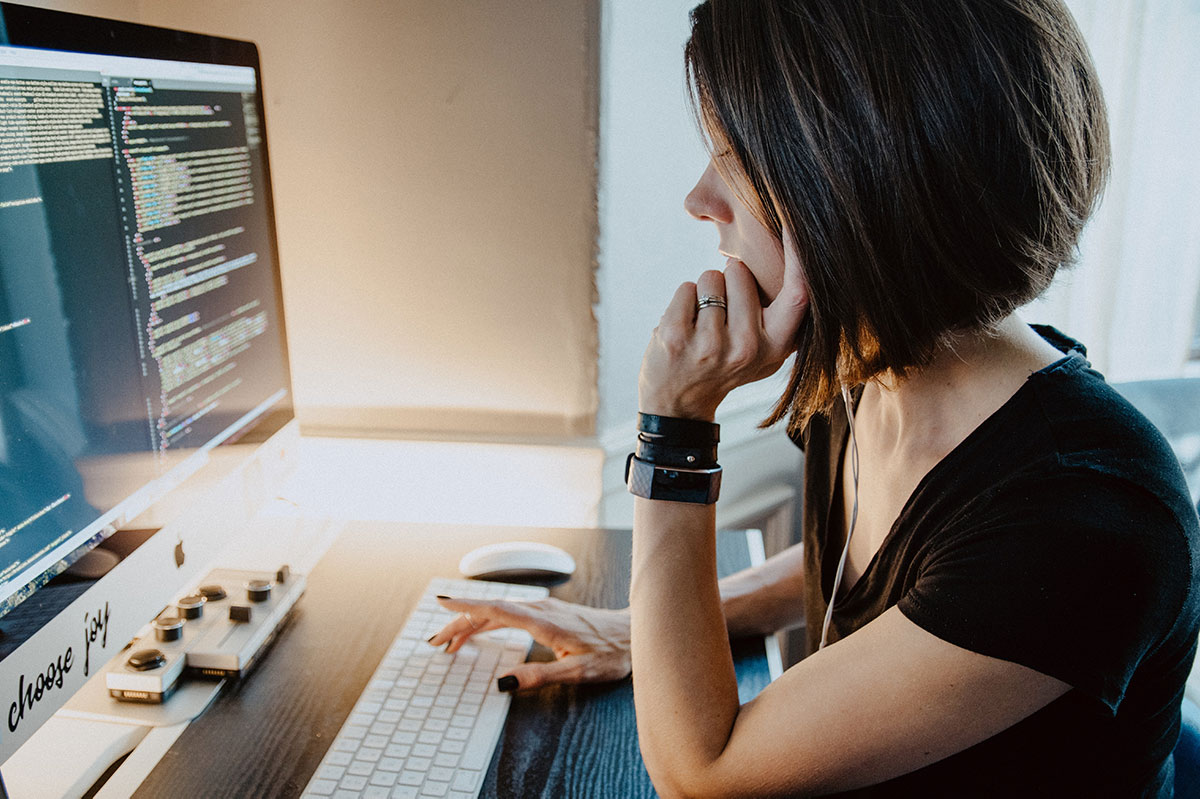
Don’t forget developers
Another pool of talent that tends to have the analytic experience and expertise in robust testing to help offset a lack of internal data science talent, are developers, especially those that are more mathematically inclined. Their experience in delivering robust applications combined with an AutoML platform that takes away most of the complexity of delivering AI solutions, with minimal input from experienced data scientists, they can deliver very effective AI products and solutions.
In Summary
So while cucumber farmers and shopkeepers are unlikely to see much benefit from driving AutoML tools, there are many people in most organisations who are well placed to pick up these technologies and start delivering value with AI and machine learning. What we see work best is training those with adjacent skills in data insights or coding. And while they should be able to be largely independent, they will need a small amount of support and guidance and this should be a key consideration in the purchasing decision, when adopting these technologies. When getting customer references, ask them about their experiences of support, how they helped through the adoption curve to get real value from AI and ML.
Get In Touch
Whether you're just starting your AI journey or looking for support in improving your existing delivery capability, please reach out.
By submitting this form, I can confirm I have read and accepted Kortical's privacy policy.