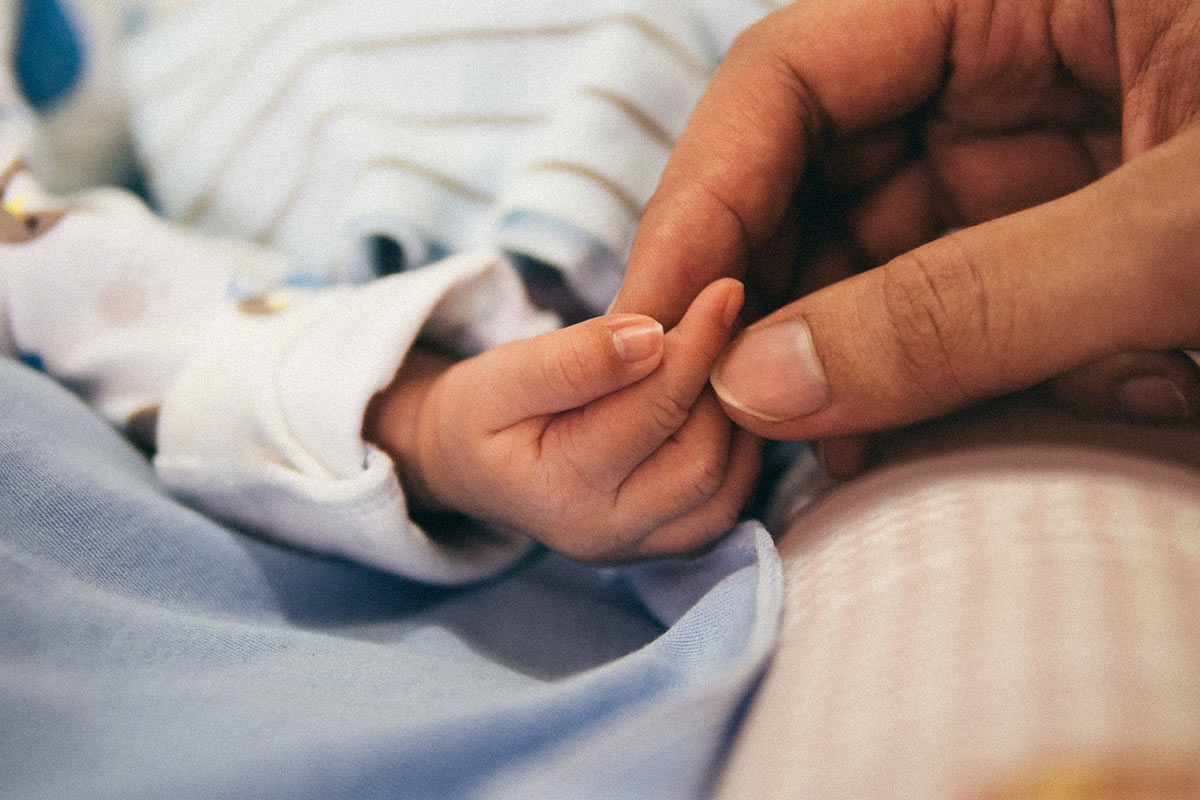
AI supply chain optimisation for platelets to reduce costs
54%LESS EXPIRES
100%LESS AD HOC TRANSPORT
06MONTH FULL DIGITAL TRANSFORMATION
A leading US Hotel chain almost always had a number of rooms which were empty. They needed to fill as many of these rooms as possible. Sending offers using traditional marketing techniques (based on segmentation) had the common issue that
Kortical built an AI solution in 4 weeks which could predict which customers to target, predict the type of room they might book and then tailor a personalised offer (determining the tier of room and level of discount to best offer in order to maximise overall revenue). This resulted in a 56% increase in revenue vs their existing campaign and 1,000% ROI on the marketing spend proven out over an 8 week pilot.
The US hotel franchise has an ever changing number of rooms which are unoccupied at various points of the year through seasonal demand and many other factors. They want to try and maximise occupancy while maximising revenue (minisming any offers/incentives needed to fill the rooms).
The problem is complex as demand fluctuates throughout the year, and the optimal incentives to book a room change from customer to customer (who are themselves price sensitive to varying degrees). We could, for example, send a customer an insufficient incentive and thereby leave the room unoccupied (losing potential revenue), but also conversely offer a generous incentive to a customer who would have taken the room at a lower discount (losing margin).
The existing system was using conventional segmented marketing to send out offers to customers, to incentivize them to fill vacant rooms. The system represented the limit of what was possible with traditional marketing techniques before you add the hyper-personalisation capable with AI.
Kortical was brought in to help solve this issue via an AI powered hyper-personalised marketing system over a 12 week project. The first 4 weeks were used to build the solution and then the remaining 8 weeks were used to pilot it live in an A/B test vs the existing system.
The objective was to increase the revenue generated by the existing marketing campaign through the use of Kortical to:
The metric being used to evaluate the system as overall revenue increase vs the existing marketing solution in an A/B test.
Our approach involved a three tier system that involved two machine learning models and an optimiser. These were combined to make a Hyper-personalised offer targeting solution.
The first model predicted which of the hundreds of thousands of customers were the most likely to require a room at a given location at a given point in time. This is a methodology taken from the retail industry, whereby those most likely to make a purchase often have the most headroom for increased spend.
The second model, run only on those customers who were selected as high propensity by the first model, predicted how likely a customer would be to book a particular room tier, given a certain offer. For each customer we would then have a set of probabilities against all combinations of room tier and offer level.
Finally, for each customer, an optimiser determined the offer we would send based on calculating the expected value 1 for each room tier and offer level and then selecting the maximum. For example, if we can see that our customer has a 50% chance of booking a $100 room with a 10% discount, the expected value would be $45. If we could see the same customer had a 35% chance of booking a $200 room with a 30% discount, the expected value would be $49, so we should offer her a bigger discount on the more expensive room.
When we first uploaded the data, Kortical data prep helped us resolve some issues with missing data such as the zip code field being blank
1 The expected value is a concept from statistics that asks “If this event was to happen a large number of times, what would be the average return I would receive?”. So a 10% chance of winning £50 would be an expected value of £5 where a 1% chance of winning £1000 would be an expected value of £10.
Kortical infers the column types, so all we needed to do was set the evaluation metric to log loss and start searching for the best solution
Tens of thousands of candidate models were generated with Korticals smart AutoML - each new candidate is a new hypothesis as to the best solution for the problem - you can see them ranked on the leaderboard
We explored some of the top candidates, examining the ROC curves and f1 tables across our permutations of discount and room tier
Finally, we run some of the top models through a business case test which allows us to test each model against the optimiser and find the model which performs the best at our downstream offer optimisation task
To get the first version live rapidly, we can use Korticals built-in google sheets plugin to pull predictions directly into a spreadsheet based app from the platform - this allows end users to consume the model predictions immediately, avoiding any app built out phase.
For the first model we created a binary classifier trained on a customer’s booking history and any demographic detail which was available. The platform trained over 15k models, maximising area under the ROC curve.
The second model was a multi-class classifier, using the same features as our binary model, which had a class mapped for each of the room tier x discount permutations. We used log loss as the metric to optimise for (as we wanted to directly use the model output as pseudo-probabilities). The platform searched through over 10k different solutions before we were happy with the final model.
The final stage, the optimiser, enumerated this matrix of probabilities from the multi-class classifier (room tier x discount) and took the maximum. This was then used to drive a marketing platform sending the best offer to the appropriate customers.
Kortical was able to have this hyper-personalised ML marketing predictions system, built, trained and deployed in 4 weeks and then prove it out over the next 8 weeks in an A/B test.
The custom offers were a resounding success, with the overall click rate improved by 10%, the revenue generated increased by 56% and the marketing ROI doubled to 1000%.
Whether you're just starting your AI journey or looking for support in improving your existing delivery capability, please reach out.
By submitting this form, I can confirm I have read and accepted Kortical's privacy policy.