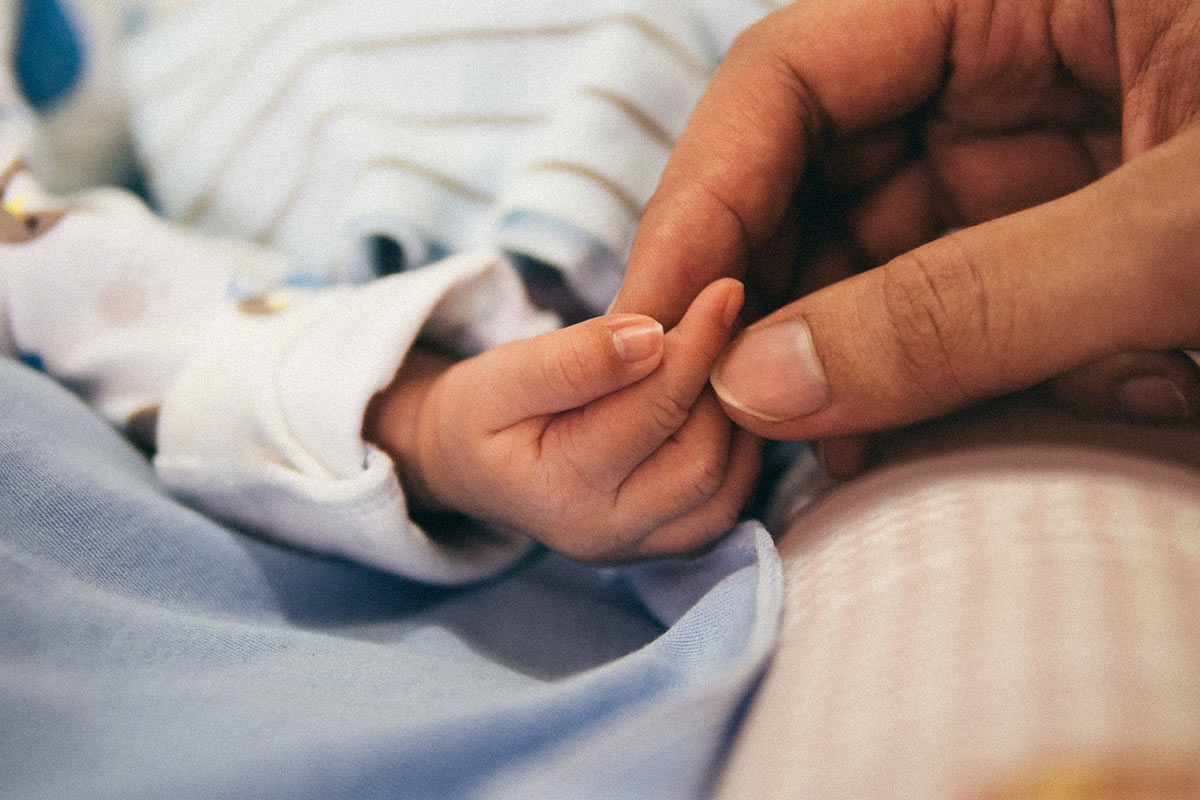
AI supply chain optimisation for platelets to reduce costs
54%LESS EXPIRES
100%LESS AD HOC TRANSPORT
06MONTH FULL DIGITAL TRANSFORMATION
Predicting vehicle failures before they happen via connected modem data with Ford
Predicting failures before they affect the customer is a holy grail for large OEMs like Ford. With cars sending a vast array of sensor data, they were keen to understand if machine learning applied to connected modem data could predict which vehicles were imminently at risk of specific equipment failures prior to a breakdown.
They engaged Kortical to apply our knowledge of artificial intelligence and predictive algorithms along with our highly efficient AI Cloud platform to help Ford prove if applying machine learning predictive maintenance to this real time data, would deliver: time and ultimately cost savings for their customers.
Ford's commercial vehicle fleets provide the backbone for many businesses, both small and large. Reducing maintenance downtime represents a huge benefit for these customers, since many of their employees cannot work while their vehicle is in for service.
The business case Kortical helped Ford solve, involved minimising the downtime of the Ford Transit vehicles, with scheduled maintenance vs reactive maintenance. By using AI applied to vehicle modem data, we were able to detect certain failures before they had fully manifested, this meant Ford could send the appropriate spare parts to the nearest dealer and preemptively book in a session.
The pay off for the customer was that instead of a vehicle being in the mechanics for 24 hours while they waited for parts to arrive, it could instead be serviced within 3 hours and save 21 hours of downtime.
If the economics made sense we could use the model for preemptive maintenance to proactively fix parts that were predicted to fail in the future but were not yet imminent. This works well for cheaper parts, where the overheads of the service are a significant portion of the costs. As well as the operational cost savings, there is less fleet downtime for the vans.
Dealers could also anticipate and schedule servicing slots more effectively, since they had an advanced warning of upcoming demand. Smart scheduling, for greater operational efficiency, was an internal major pain point this predictive maintenance technology could unlock.
While the connected vehicle data already contained many hand crafted features known as DTCs (Diagnostic Trouble Codes), when used in isolation these DTCs produced an unacceptably high false positive rate. This is because a DTC becomes activated given certain conditions in the engine based on sensor data but it is not able to understand the broader context, such as other issues which could be causing these readings. For example certain issues with the battery will cause certain other DTCs to fire incorrectly, since low voltage causes conditions similar to other failure modes.
By using machine learning to interpret the full set of DTC data and also to take into account broader contexts such as recent repairs, build numbers and vehicle metadata, we were able to achieve a much lower false positive rate for any given issue than was achievable simply using the DTC alone. For a subset of 22% of failure cases, we were highly accurate 10 days in advance of the failure fully manifesting and so enabling the predictive maintenance business case.
The business impact across customers for this one specific part was just over 122,000 hours of downtime saved. Ford anticipates by being able to put a predictive maintenance program in place, this will lead to a halo effect in the order of $millions since reliability and lower maintenance costs are a huge selling point when making commercial fleet purchasing decisions.
Full details of the case study in the webinar below with Ford, Kortical & IDE (who provided the grant to undertake this research).
Whether you're just starting your AI journey or looking for support in improving your existing delivery capability, please reach out.
By submitting this form, I can confirm I have read and accepted Kortical's privacy policy.